The future of Radiology in the Era of Artificial Intelligence
With Curtis P. Langlotz, MD, PhD, Stanford University
- Time:2024-10-15, 12.00-13.00 (standing lunch 13.00-13.45)
- Location: Nobel Forum, Nobels väg 1, Karolinska Institutet
- Language: English
- Participating: Curtis P. Langlotz
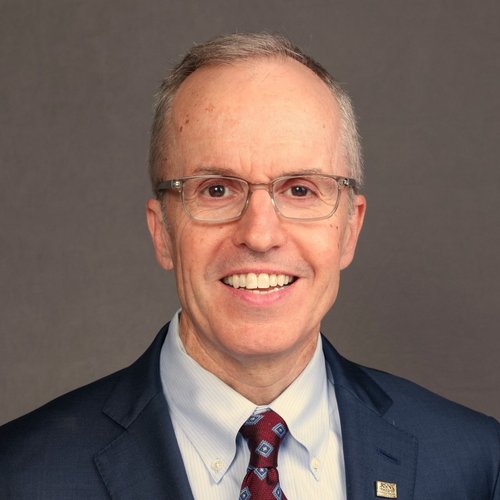
Last day to reply: October 8. Sign-up: https://www.kth.se/form/future-of-radiology-langlotz
Curtis Langlotz’s lecture will be of interest to radiologists, engineers in academia and industry, AI researchers and students.
By attending, you will review the origins of artificial intelligence (AI) for medical imaging, predict how AI will change the practice of radiology using current examples, and assess the shortcomings of AI that will limit its effect on the radiology work force. After the lecture you will be able to:
- Understand the origins of AI and machine learning and their application to medical imaging
- Predict how artificial intelligence will change the practice of radiology using current examples
- Describe how large language models will affect patient engagement with radiology
- Assess the shortcomings of artificial intelligence that may limit its applicability
Abstract
Artificial intelligence (AI) is an incredibly powerful tool for building computer vision systems that support the work of radiologists. Over the last decade, artificial intelligence methods have revolutionized the analysis of digital images, leading to high interest and explosive growth in the use of AI and machine learning methods to analyze clinical images and text. These promising techniques create systems that perform some image interpretation tasks at the level of expert radiologists. Deep learning methods are now being developed for image reconstruction, imaging quality assurance, imaging triage, computer-aided detection, computer-aided classification, and radiology report drafting. The systems have the potential to provide real-time assistance to radiologists and other imaging professionals, thereby reducing diagnostic errors, improving patient outcomes, and reducing costs. We will review the origins of AI and its applications to medical imaging and associated text, define key terms, and show examples of real-world applications that suggest how AI may change the practice of radiology. We will also review key shortcomings and challenges that may limit the application of AI to radiology.
About Curtis P. Langlotz, MD, PhD
Dr. Langlotz is Professor of Radiology, Medicine, and Biomedical Data Science, and Senior Associate Vice Provost for Research at Stanford University. He also serves as Director of the Center for Artificial Intelligence in Medicine and Imaging (AIMI Center), which comprises over 150 faculty at Stanford who conduct interdisciplinary machine learning research to promote health. Dr. Langlotz’s laboratory develops machine learning methods to detect disease and eliminate diagnostic errors. He has led many national and international efforts to improve medical imaging, including the RadLex terminology standard and the Medical Imaging and Data Resource Center (MIDRC), a U.S. national imaging research resource.